Full Tensor Migration is a fast, robust, unconstrained inversion method. It serves as a means to separate signal in final processed tensor data producing meaningful depth interval anomaly maps.
Traditional methods separate signal according to frequency content but often suffer from leakage of bandwidth, thus making the exercise to be quite troublesome. For example, high density shallow sources often generate high signal strength with high amplitude anomalies. Filtering such signal is challenging when trying to extract signature from deeper more subtle geology; one typically ends up removing more signal than is desired and so achieve a less than expected result.
Full Tensor Migration overcomes this challenge. It is a fast, robust unconstrained inversion that quickly identifies depth relationships in the data. Final processed tensor signal is migrated through a density field to identify depth.
We initially transform the final processed tensor signal to a density field under the assumption that all sources reside at a depth equal to the observation surface. This density field then undergoes an iterative automated forward modelling exercise to identify depth sensitivity. Each modelled output is examined by plotting it’s signal intensity against the modelled depth. The signal intensity is identified as the standard deviation from an Invariant representation of the tensor. The curve peaks point to greatest sensitivity; and, as for example, as shown in the chart below, we see two such peaks, 2km depth and again at 11km depth.
This clearly tells us that the FTG data on this particular survey shows sensitivity to sources arising from these two depth intervals meaning that interpretative work can now proceed with high confidence
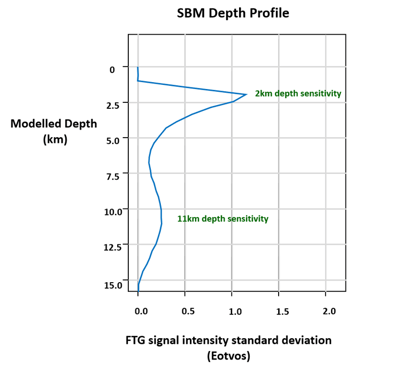
An additional key benefit from Full Tensor Migration is that it serves to ‘clean’ final processed tensor data, i.e. remove the ‘orange peel’ effect that so often makes for difficult visuals of gravity gradient data. This ‘orange peel’ effect is characterised by a high frequency, low amplitude signal and generally considered as residual processing noise. The impact is profound as it can severely limit a meaningful interpretation.
The survey data example shown below is a prime example of how Full Tensor Migration cleans final processed tensor data.
The image on the left is a final processed terrain corrected Tzz. The survey was acquired with 2km line spacing over a large basinal area. The high amplitude anomaly patterns on the SW and NE margins are associated with shallowing basement; the lower amplitude anomalies in the centre are associated with geological sources residing at depths of 2 to 4km. The final processed Tzz is clearly contaminated with residual noise making it difficult to interpret the underlying anomaly pattern.
The middle image is the result from the Full Tensor Migration workflow. We clearly see a significant improvement in signal clarity and that many of the anomaly patterns are now much more coherent. Numerous trends are evident from those paralleling the basin margins to localised E-W trends in the basin centre.
The right hand image is the residual field, i.e. the signal left over from the Migration workflow. Full Tensor Migration clearly identified and extracted a stable, highly coherent, high S/N anomaly field. The residual image captures the residual noise that yields the ‘orange peel’ effect in standard processing of tensor data
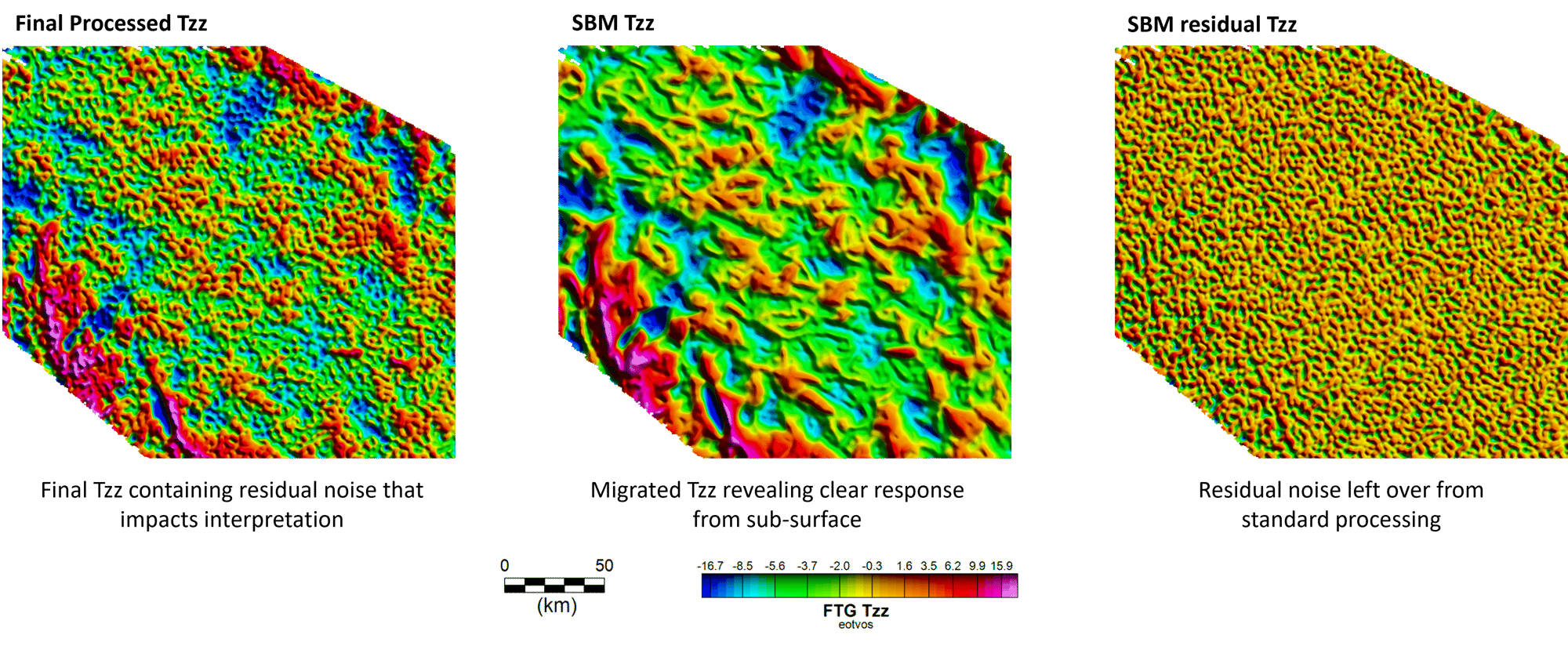